모델의 성능이 언제까지나 유효한가? No
"Past performance is no guarantee of future results."
"No model lives forever, but the speed of decay varies."
실 사용사례에서는 데이터가 계속 달라지기 때문에 모델의 성능이 점점 떨어지는 현상을 발생시킨다.
모델의 quality를 판별하는 데에 사용하는 것은 Accuracy, Mean Error Rate, or some downstream business KPI such as click-through rate (꼭 F1 score 만 사용하는 것은 아님)
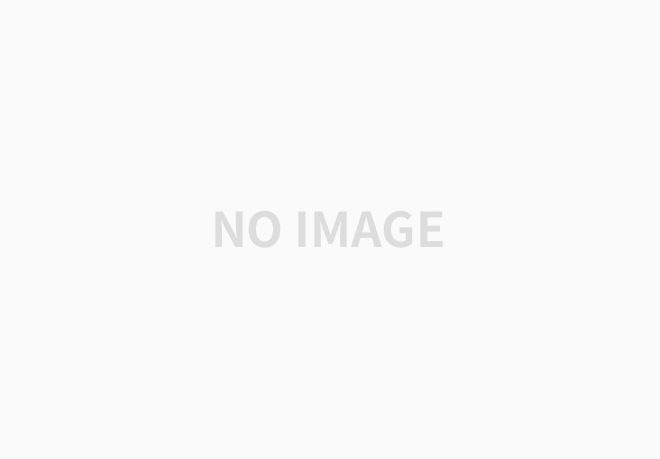
다양한 모델의 quality 판별을 통해서 검토를 한 결과 성능이 저하게 된다는 것을 "Model decay" 라고 한다.
반응형
'MLOps > 이론' 카테고리의 다른 글
MLOps Level 1 : ML pipeline automation (0) | 2024.03.23 |
---|---|
MLOps Level 0 (0) | 2024.03.23 |
Model Decay의 이유(1) : Data Drift, Training-serving Skew (0) | 2024.02.23 |
ML 서비스 개발 순서 (0) | 2024.02.22 |
MLOps를 위한 기초관점 (0) | 2024.02.20 |