그렇다면 이런 이유에는 어떤 것들이 있을까?
1. Data drift - feature drift, population, or covariate shift
데이터가 지속적으로 변경되고, 그에 따라 변수들의 분포가 의미있게 달라지게 된다.(과거 형태의 데이터에는 유효한 모델!)
연구용 ML에서는 같은 데이터(주로 benchmark)를 사용하기 때문에 문제가 되는 부분이 아니지만 프로덕션 ML에서는 중요한 부분이라고 생각된다.
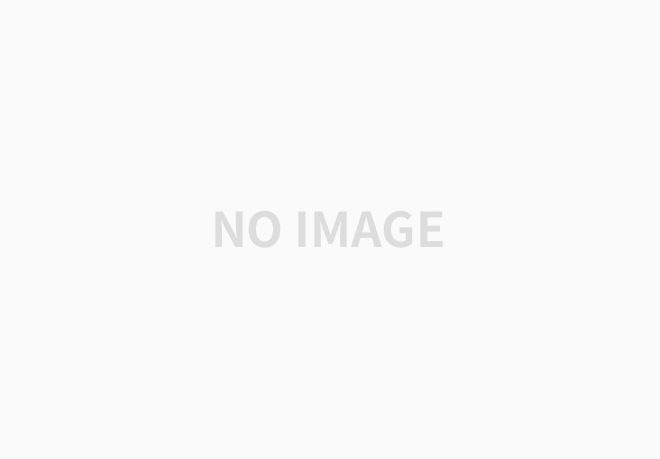
2. Training-serving skew
학습한 데이터가 너무 깔끔해서(artificially constructed or cleaned data) 실제로 모델 사용 과정에서 받는 데이터셋(Production data)과 차이가 발생하는 경우
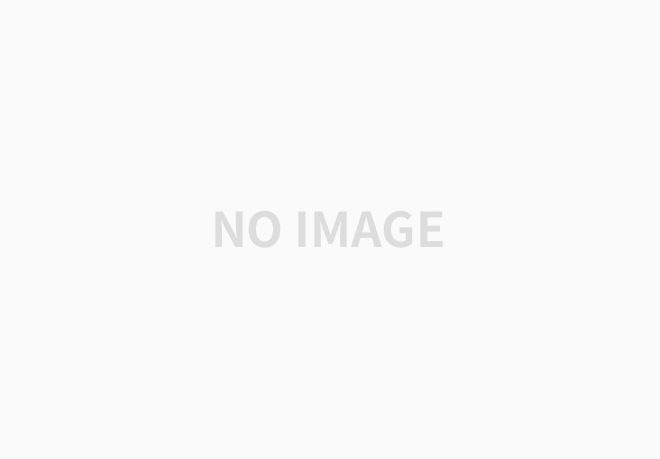
이럴 경우에는 주로 모델을 실제 데이터를 활용해서 추가 학습하는 방식으로 문제를 해결.
In most cases of training-serving skew, the model development has to continue.
3. Concept drift(2에서 추가적으로 다룰 예정)
모델의 학습된 패턴이 변질될 경우에 발생
Concept drift occurs when the patterns the model learned no longer hold
Concet drift means that the statistical properties of the target variable, which the model is trying to predict, change over time in unforeseen ways
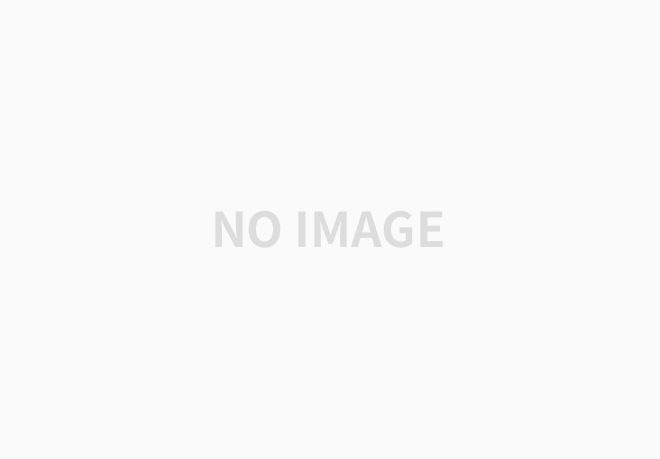
반응형
'MLOps > 이론' 카테고리의 다른 글
MLOps Level 1 : ML pipeline automation (0) | 2024.03.23 |
---|---|
MLOps Level 0 (0) | 2024.03.23 |
프로덕션 ML 시스템의 특징(Model Decay) (0) | 2024.02.22 |
ML 서비스 개발 순서 (0) | 2024.02.22 |
MLOps를 위한 기초관점 (0) | 2024.02.20 |